Treatments for relapsing and/or refractory multiple myeloma. A health technology assessment
Health technology assessment
|Published
The Norwegian Institute of Public Health (NIPH) was commissioned by the RHA forum to perform a full health technology assessment on the pharmacological treatment of multiple myeloma.
Key message
In the first version of this report the sources for the prices of Ixasomib and Isatuximab accidentally reversed in table 19, page 96. This was corrected on april 25th 2023.
In the PDF file, prices are redacted for reasons of confidentiality for the manufacturer. In the text below, the hidden figures appear as X.
Multiple myeloma is the second most common type of blood cancer in Norway, with approximately 450 new cases diagnosed annually. There is currently no cure for multiple myeloma, so the goals of treatment are to prolong life, to achieve as strong a response as possible without unacceptable side effects, and to maintain the patient’s quality of life at as high a level as possible throughout treatment.
We carried out a health technology assessment of different treatment regimens for patients with multiple myeloma who are refractory to treatment, or experience relapse. We included data for five outcomes: overall survival, quality of life, severe adverse events, progression-free survival and discontinuation due to adverse events, with overall survival being our main primary outcome. Data were not available for all treatment regimens and outcomes. We inferred that there is not a single treatment regimen that is superior with respect to all outcomes. The following six triplet combinations are examples of treatment regimens relevant for non-refractory patients in a Norwegian setting, with clearly favorable hazard ratios for overall survival, that are also ranked highly with respect to other outcomes:
- [EP + d]: elotuzumab (E), pomalidomide (P) and dexamethasone (d)
- [IsP + d]: isatuximab (Is), pomalidomide (P), and dexamethasone (d)
- [DK + d]: daratumumab (D), carfilzomib (K), and dexamethasone (d)
- [KR + d]: carfilzomib (K), lenalidomide (R), and dexamethasone (d)
- [DR + d]: daratumumab (D), lenalidomide (R), and dexamethasone (d)
- [DV + d]: daratumumab (D), bortezomib (V), and dexamethasone (d)
The health economic analysis relied on a partitioned survival analysis to estimate total costs (in NOK), health gains (in quality-adjusted life years; QALYs), and incremental cost-effectiveness ratios (ICER) for treatments of interest. We grouped treatments into three separate groups, each based on the relevant reference treatment in order to perform the analysis. We used hazard ratios from the network meta-analysis to inform the overall and progression-free survival curves used in the model. The results of the health economic analyses were:
- In the [R + d] group, [R + d] had costs of NOK X, with 2.90 QALYs gained. Only two other treatments were not dominated by other treatments: [IR + d] had costs of NOK X, 3.82 QALYs, and an ICER of NOK X compared to [R + d]. [DR + d] had costs of NOK X, 4.31 QALYs, and an ICER of NOK X compared to [IR + d].
- In the [V + d] group, [V + d] had costs of NOK X and 2.24 QALYs. [DV + d] was the only other treatment that was not dominated by other treatments, with costs of NOK X, 63 QALYs, and an ICER of NOK X compared to [V + d].
- In the [P + d] group, [P + d] had costs of X, and 0.81 QALYS. [EP + d] was the only other treatment that was not dominated by other treatments, with costs of NOK X, 1.39 QALYs, and an ICER of NOK X compared to [P + d].
It is important to note the substantial uncertainty in the evidence underlying these results. We suggest putting more weight on comparisons from direct estimates from randomized trials, when possible. The results of the cost-effectiveness analysis should be considered highly uncertain because of lack of access to patient level data and the large degree of uncertainty in the network meta-analysis results which informed our work. Our results cannot be used to determine the best treatment sequencing among relapsed and/or refractory multiple myeloma patients.
Summary
Background
Multiple myeloma is the second most common type of blood cancer, with approximately 450 new cases diagnosed annually in Norway. The median age at diagnosis is approximately 70 years, and incidence is rare among individuals under age 30. Multiple myeloma affects plasma cells in the bone marrow. Because there is currently no cure for multiple myeloma, the goal of treatment is to achieve as strong a response as possible without unacceptable side effects, and to maintain the patient’s quality of life at as high a level as possible throughout treatment.
Objective
To determine the clinical efficacy, safety, and cost-effectiveness of disease modifying treatments for relapsed and/or refractory multiple myeloma (RRMM) in a Norwegian context.
Efficacy and safety
Method
We have systematically collected and reviewed the evidence for clinical efficacy and safety for disease modifying treatments for relapsed and/or refractory multiple myeloma according to the PRISMA rules. We identified relevant publications from randomised, controlled trials (RCTs) through systematic reviews from our previous mapping review, as well as through systematic searches. The inclusion criteria were individuals over 18 years diagnosed with multiple myeloma, who either were refractory to at least one previous line of treatment or had experienced one or more relapses. The treatment (intervention) was any of the drugs listed below, alone or in combination with each other, and/or with a glucocorticosteroid such as dexamethasone, compared with any intervention-drug alone, or in combination with each other, or in combination with other drugs.
Treatments named in the commission and listed in the project plan (protocol):
Abb. |
Full drug name |
D |
Daratumumab |
E |
Elotuzumab |
F |
Panobinostat (Farydak) |
I |
Ixazomib |
Is |
Isatuximab |
K |
Carfilzomib |
P |
Pomalidomide |
R |
Lenalidomide (Revlimid) |
V |
Bortezomib (Velcade) |
The primary outcomes were overall survival, health related quality of life, and severe adverse events, with overall survival being our main primary outcome. Secondary outcomes were progression free survival, adverse events, and discontinuation due to adverse events. We used the critical appraisal of one systematic review from which we included several studies; two researchers critically appraised the remaining included studies. All outcomes were analysed by component network meta-analyses. We present results for the treatment regimens relevant for Norway, as determined by the Norwegian guideline for multiple myeloma, with results for all included treatment regimens in the appendix. We assessed the certainty of evidence for all outcomes using the GRADE approach (Grading of Recommendations Assessment, Development and Evaluation), expressing the certainty as high, moderate, low, or very low, depending on the level of confidence we have in the effect estimates.
Results
We included in total 72 articles from 50 RCTs that studied the effects of various treatment regimens containing one to three disease modifying drugs. We performed component network meta-analyses on up to 34 randomised, controlled trials, comprising 12 873 randomized patients, and 31 treatments.
The radar plots below illustrate the overall safety and efficacy of each treatment regimen across all outcomes. Each individual radar plot presents the available P-scores for the different outcomes, for each treatment regimen, as a polygon (shaded). A P-score expresses the mean extent of certainty that a given treatment regimen is superior to all other regimens included in the underlying meta-analysis (i.e., with respect to a single outcome such as overall survival). They are informally interpreted as the probability that a treatment is “best”. A treatment with a higher P-score (closer to 100%) could be interpreted to be superior (i.e., longer survival, better quality of life, fewer severe adverse events, longer progression-free survival or fewer discontinuations due to adverse events) to a treatment with a lower P-score (closer to 0%). In the radar plots, treatment regimens with polygons with larger areas tend to be superior to those with smaller areas. However, this interpretation can be misleading because data was not available for all treatments and outcomes: it is therefore possible for a highly effective treatment to have a polygon with small area due to a lack of data. When comparing results for different treatment regimens, one should be careful not to interpret effect based solely on polygon area.
We inferred that there is not a single treatment regimen that is superior with respect to all outcomes. Radar plots for the double combination [P + d] exemplify treatment regimens that have polygon with large area. This would indicate better efficacy and safety than treatment regimens with smaller area polygons, e.g., [DR + d]. However, when looking closer at the individual P-scores, we find that [P + d] have lower P-score for overall survival than [DR + d]. As such, we would expect longer survival by treatment with [DR + d] than [P + d]. While radar plots may be useful for understanding tradeoffs between efficacy and safety, they should not be interpreted in isolation. Furthermore, the radar plots do not reflect assessments of the certainty of evidence or results of the health economic analysis. We assessed the certainty of evidence (GRADE) for one treatment being better than another, to be mainly low or very low, with a few exceptions.
The six triplet combinations [EP + d], [IsP + d], [DK + d], [KR + d], [DR + d] and [DV + d] are examples of treatment regimens relevant for non-refractory patients that have clearly favorable hazard ratios for overall survival, that also are ranked highly with respect to other outcomes.
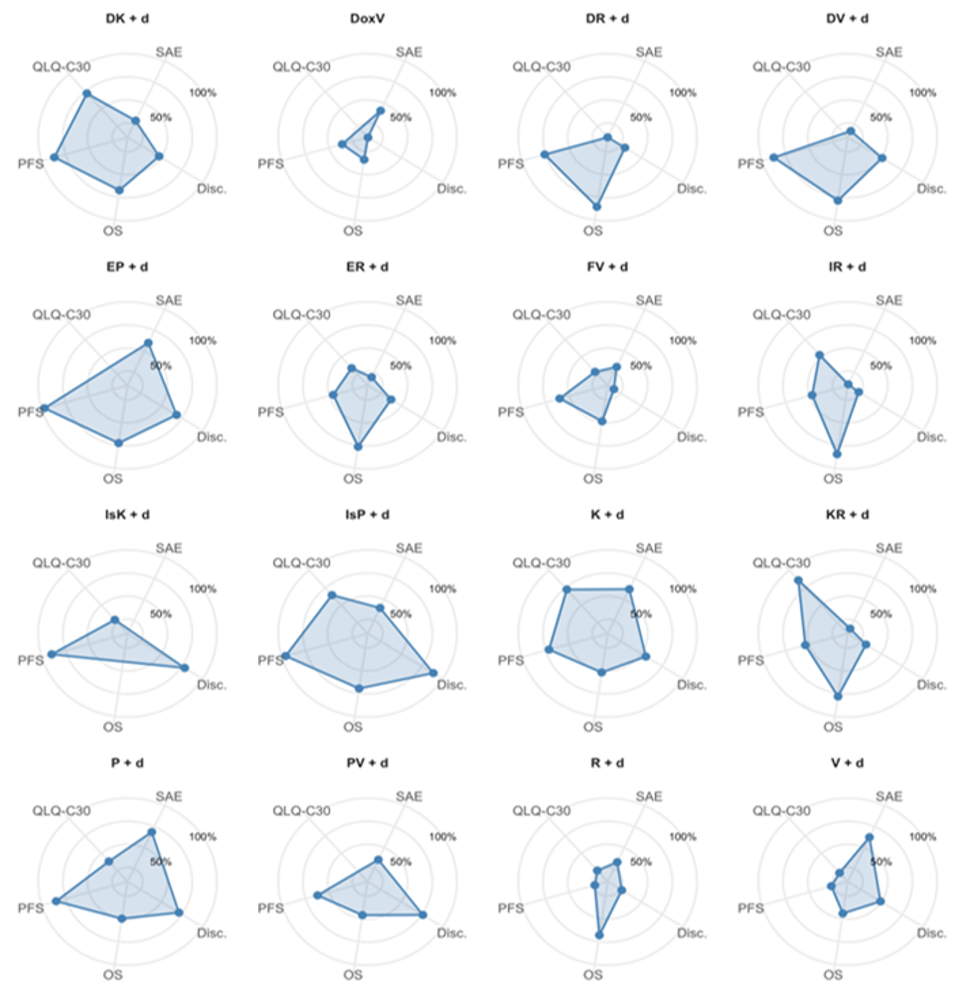
Radar plot of treatment regimens relevant for non-refractory patients
Radar plots show treatment regimens relevant to Norwegian clinical practice. D: daratumumab, d: dexamethasone, Disc.: discontinuation due to adverse events (risk ratio), Dox: doxorubicin, E: elotuzumab, F: panobinostat, I: ixazomib, Is: isatuximab, K: carfilzomib, OS: overall survival (hazard ratio), P: pomalidomide, PFS: progression-free survival (hazard ratio), QLQ-C30: quality of life (difference in mean score), R: lenalidomide, SAE: severe adverse events (incidence rate ratio), V: bortezomib. The radar plots summarize relative efficacy and safety but do not reflect assessments of the certainty of evidence or results of the health economic analysis.
Health economic evaluation
Method
We conducted a cost-utility analysis of 13 treatments for patients with relapsed and/or refractory multiple myeloma in which health effects were measured in quality-adjusted life-years (QALYs), costs in Norwegian kroner, and results were presented as incrementals cost-effectiveness ratios (ICERs). We chose to use a partitioned survival analysis model implemented in TreeAge to perform the analysis. Partitioned survival analyses are frequently used to model the cost-effectiveness of cancer treatments because Kaplan-Meier plots of overall and progression-free survival curves from clinical trials can be used to track patients through three health states: Progression-free, Progressed, and Dead.
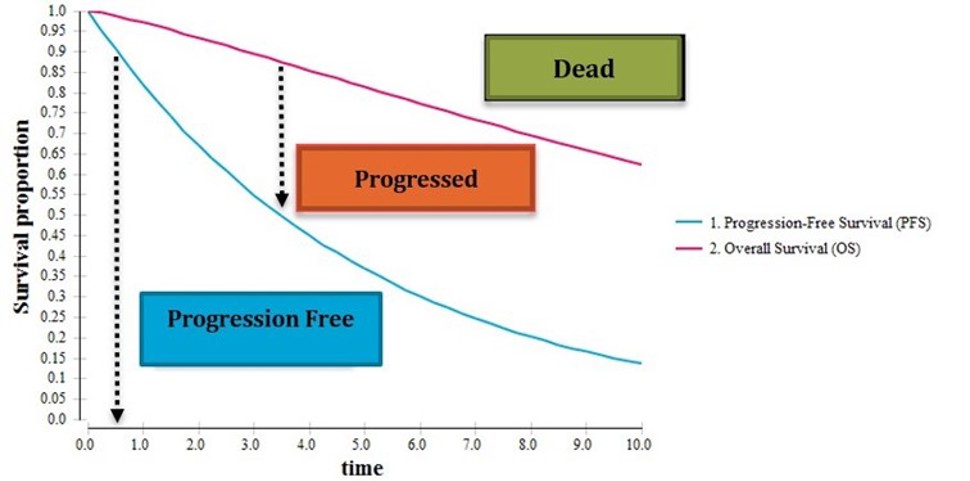
Survival Curves and Health States in Partition Survival Analysis
OS – PFS = Progressed, PFS = Progression Free, OS = Alive (Progressed + Progression Free)
Without access to patient level trial data it is not possible to generate well-fitted survival curves for each treatment in the model. Instead, we used a technique, common in cost-effectiveness analyses, in which a survival curve is generated for the comparator treatment in the analysis and the corresponding curves for interventions of interest are generated by applying the relevant hazard ratios from a meta-analysis to the comparator’s survival curve. As the hazard ratios for overall and progression-free survival were taken from the network meta-analysis in the clinical effect section of this report, there was not a “comparator” in the normal sense. In a network meta-analysis any treatment can be designated as a “reference treatment” since the matrix of results generated by the analysis provides hazard ratios for each intervention relative to all other interventions. Based on expert advice, we subdivided our economic model into three treatment groups, each based on one of three reference treatments: lenalidomide (Revlimid) + dexamethasone [R + d], bortezomib (Velcade) + dexamethasone [V + d], and pomalidomide + dexamethasone [P + d].
The [R + d] group included: [R + d], [DR + d], [RK + d], [ER + d], and [IR + d].
The [V + d] group included: [V + d], [DK + d], [K + d], [FV + d], and [DV + d].
The [P + d] group included: [P + d], [EP + d], and [IsP + d].
Costs for the analysis included: 1) cost of medications, 2) time costs for pharmacy and nursing staff for preparation and administration of medications given by injection or infusion, 3) time costs for doctor visits and tests at regular check-ups, and 4) patients’ travel and time costs associated with treatment. We were unable to include costs of severe adverse events, as they were not reported consistently in published trial results, but as these are quite small in relation to medication costs, they would not have resulted in meaningful changes in the results. To account for uncertainty associated with the variables included in the model (hazard ratios, utility values for capturing quality-of-life and treatment costs) we ran the model as a probabilistic sensitivity analysis with 10,000 random draw Monte Carlo iterations. The model also allowed us to calculate absolute shortfall, the variable used to determine the severity of a disease. We also conducted one-way sensitivity analysis to determine which variables had the largest impact on the results.
Results
We report the cost-effectiveness results for treatments in each reference group that were not dominated by another treatment (the sums given as X because of Norwegian confidentiality law). A treatment is considered dominated if it has a higher total cost and lower health effect than another treatment. In the [R + d] group, [R + d] had costs of NOK X, with 2.90 QALYs gained. Only two other treatments were not dominated by other treatments: [IR + d] had costs of NOK X, 3.82 QALYs, and an ICER of NOK X compared to [R + d]. [DR + d] had costs of NOK X, 4.31 QALYs, and an ICER of NOK X compared to [IR + d]. In the [V + d] group, [V + d] had costs of NOK X and 2.24 QALYs. [DV + d] was the only other treatment that was not dominated by other treatments, with costs of NOK X, 3.63 QALYs, and an ICER of NOK X compared to [V + d]. In the [P + d] group, [P + d] had costs of X, and 0.81 QALYS. [EP + d] was the only other treatment that was not dominated by other treatments, with costs of NOK X, 1.39 QALYs, and an ICER of NOK X compared to [P + d].
In addition to providing cost-effectiveness results, the health economic analysis provided estimates of average absolute shortfall for treatments in each of the reference groups. The values were similar across groups and ranged from 12.46 to 14.95 lost healthy life-years.
Discussion
Efficacy and safety
Our report is limited in having few RCTs for each treatment regimen. Because the included studies defined disconnected networks, we had to use component network meta-analysis, and were unable to formally test the assumption that the treatments can be modelled in this way. Including immature survival data in our analysis may have introduced bias in our report due to selective reporting of findings. It is likely that more mature data would lead to different meta-analysis results and more certain judgements about the evidence.
Most of the included RCTs are international studies, predominately conducted in North America and Europe. However, our overall survival results for both [IR + d] and [DV + d] are based on one small Chinese study and one large international study, where there were differences in the type of treatment offered to patients who progressed, making it difficult to interpret the findings. There were also ethnic and other differences in the study populations. There have been reported ethnic differences regarding incidence of multiple myeloma and aggressiveness of disease. In addition, ethnicity has also been shown to affect drug response in cancer treatment in some cancers. However, we do not know to what extent this does or does not apply to RRMM.
Ideally, future studies should directly compare the more effective triple regimens, rather than using less effective double regimens as controls. It may not be possible to power superiority trials comparing treatments of very similar effectiveness, but non-inferiority trials may be a useful approach when relatively small effect sizes are expected.
Health economic evaluation
There is a great deal of uncertainty connected with the results of the health economic analysis. Some of this uncertainty reflects the fact that we needed to rely on the results of the component network meta-analysis performed for the clinical effect section of this report to perform the health economic analysis. The sources of uncertainty in the network meta-analysis results have been explained in the discussion of clinical effects.
Additional uncertainty in the cost-effectiveness analysis can be the result of the methods we used to derive the underlying survival curves for the reference treatments. The lack of access to patient level data meant that we were unable to account correlation between progression-free and overall survival. This is particularly important because it limits our ability to accurately fit the portions of the survival curves beyond what is captured in the trial period. To the extent that we were unable to accurately account for dose reductions during treatment, our results may over-estimate treatment costs.
Conclusions
It is not possible to draw clear, brief conclusions for several reasons including a high degree of uncertainty across most results, the need to consider different outcomes simultaneously, and different considerations across subgroups of patients (e.g., those who are refractory to different drugs). We infer that there is no single treatment regimen that is superior with respect to all outcomes. The six triplet combinations [EP + d], [IsP + d], [DK + d], [KR + d], [DR + d] and [DV + d] are examples of treatment regimens relevant for non-refractory patients that have clearly favorable hazard ratios for overall survival, that also are ranked highly with respect to other outcomes. However, it is important to note the substantial uncertainty in the evidence underlying these results. We suggest putting more weight on comparisons from direct estimates from randomized trials, where they exist.
Cost-effectiveness results need to be viewed in the context of each reference group, as comparisons of treatments were not made across reference groups. In total, only seven treatments were not dominated by other treatments. [R + d], [IR + d] and [DR + d] in the [R + d]-group; [V + d] and [DV + d] in the [V +d]-group; and [P + d] and [EP + d] in the [P + d]-group. The ICERs for these treatments ranged from NOK X to NOK X. Absolute shortfall for patients with RRMM probably ranges from 12 to 15 healthy life-years lost. There is a high degree of uncertainty in the cost-effectiveness results.